Note
Go to the end to download the full example code
Axis scales#
By default Matplotlib displays data on the axis using a linear scale.
Matplotlib also supports logarithmic scales, and other less common
scales as well. Usually this can be done directly by using the
set_xscale
or set_yscale
methods.
import matplotlib.pyplot as plt
import numpy as np
import matplotlib.scale as mscale
from matplotlib.ticker import FixedLocator, NullFormatter
fig, axs = plt.subplot_mosaic([['linear', 'linear-log'],
['log-linear', 'log-log']], layout='constrained')
x = np.arange(0, 3*np.pi, 0.1)
y = 2 * np.sin(x) + 3
ax = axs['linear']
ax.plot(x, y)
ax.set_xlabel('linear')
ax.set_ylabel('linear')
ax = axs['linear-log']
ax.plot(x, y)
ax.set_yscale('log')
ax.set_xlabel('linear')
ax.set_ylabel('log')
ax = axs['log-linear']
ax.plot(x, y)
ax.set_xscale('log')
ax.set_xlabel('log')
ax.set_ylabel('linear')
ax = axs['log-log']
ax.plot(x, y)
ax.set_xscale('log')
ax.set_yscale('log')
ax.set_xlabel('log')
ax.set_ylabel('log')
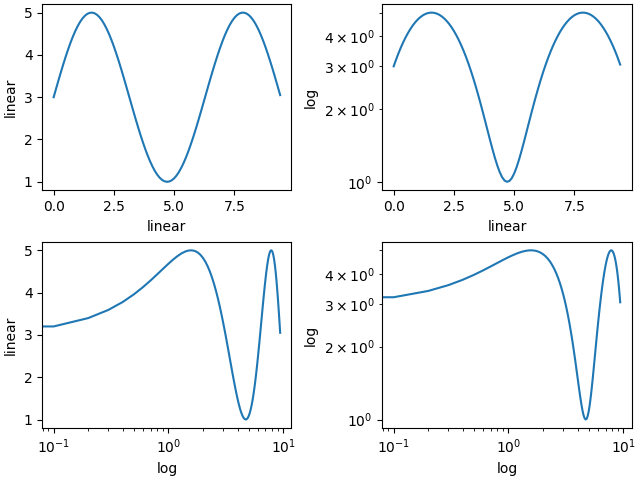
loglog and semilogx/y#
The logarithmic axis is used so often that there are a set
helper functions, that do the same thing: semilogy
,
semilogx
, and loglog
.
fig, axs = plt.subplot_mosaic([['linear', 'linear-log'],
['log-linear', 'log-log']], layout='constrained')
x = np.arange(0, 3*np.pi, 0.1)
y = 2 * np.sin(x) + 3
ax = axs['linear']
ax.plot(x, y)
ax.set_xlabel('linear')
ax.set_ylabel('linear')
ax.set_title('plot(x, y)')
ax = axs['linear-log']
ax.semilogy(x, y)
ax.set_xlabel('linear')
ax.set_ylabel('log')
ax.set_title('semilogy(x, y)')
ax = axs['log-linear']
ax.semilogx(x, y)
ax.set_xlabel('log')
ax.set_ylabel('linear')
ax.set_title('semilogx(x, y)')
ax = axs['log-log']
ax.loglog(x, y)
ax.set_xlabel('log')
ax.set_ylabel('log')
ax.set_title('loglog(x, y)')
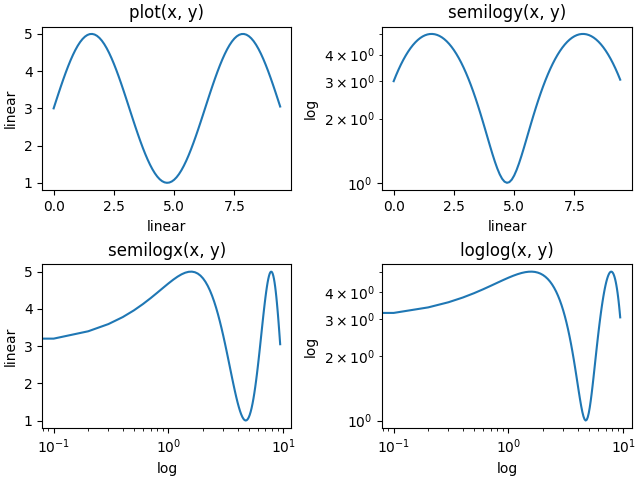
Other built-in scales#
There are other scales that can be used. The list of registered
scales can be returned from scale.get_scale_names
:
print(mscale.get_scale_names())
['asinh', 'function', 'functionlog', 'linear', 'log', 'logit', 'mercator', 'symlog']
fig, axs = plt.subplot_mosaic([['asinh', 'symlog'],
['log', 'logit']], layout='constrained')
x = np.arange(0, 1000)
for name, ax in axs.items():
if name in ['asinh', 'symlog']:
yy = x - np.mean(x)
elif name in ['logit']:
yy = (x-np.min(x))
yy = yy / np.max(np.abs(yy))
else:
yy = x
ax.plot(yy, yy)
ax.set_yscale(name)
ax.set_title(name)
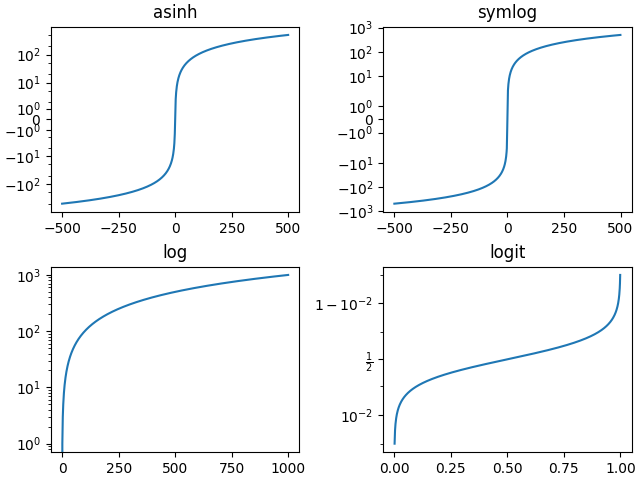
Optional arguments for scales#
Some of the default scales have optional arguments. These are
documented in the API reference for the respective scales at
scale
. One can change the base of the logarithm
being plotted (eg 2 below) or the linear threshold range
for 'symlog'
.
fig, axs = plt.subplot_mosaic([['log', 'symlog']], layout='constrained',
figsize=(6.4, 3))
for name, ax in axs.items():
if name in ['log']:
ax.plot(x, x)
ax.set_yscale('log', base=2)
ax.set_title('log base=2')
else:
ax.plot(x - np.mean(x), x - np.mean(x))
ax.set_yscale('symlog', linthresh=100)
ax.set_title('symlog linthresh=100')
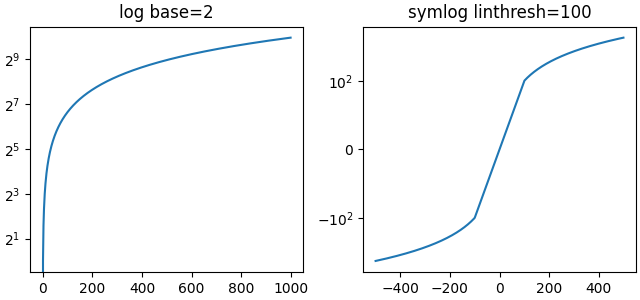
Arbitrary function scales#
Users can define a full scale class and pass that to set_xscale
and set_yscale
(see Custom scale). A short cut for this
is to use the 'function' scale, and pass as extra arguments a forward
and
an inverse
function. The following performs a Mercator transform to the y-axis.
# Function Mercator transform
def forward(a):
a = np.deg2rad(a)
return np.rad2deg(np.log(np.abs(np.tan(a) + 1.0 / np.cos(a))))
def inverse(a):
a = np.deg2rad(a)
return np.rad2deg(np.arctan(np.sinh(a)))
t = np.arange(0, 170.0, 0.1)
s = t / 2.
fig, ax = plt.subplots(layout='constrained')
ax.plot(t, s, '-', lw=2)
ax.set_yscale('function', functions=(forward, inverse))
ax.set_title('function: Mercator')
ax.grid(True)
ax.set_xlim([0, 180])
ax.yaxis.set_minor_formatter(NullFormatter())
ax.yaxis.set_major_locator(FixedLocator(np.arange(0, 90, 10)))
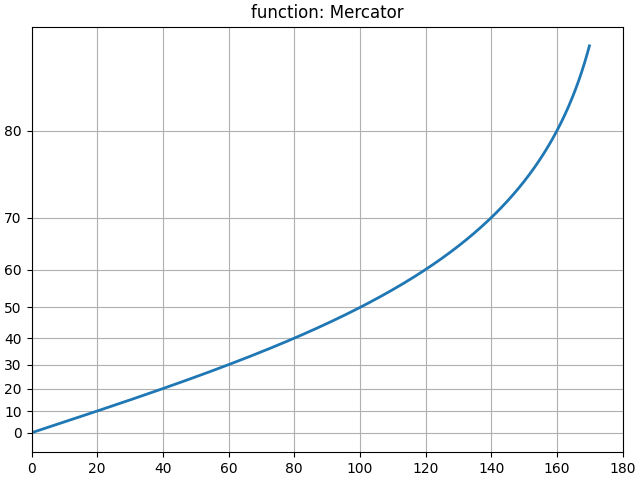
What is a "scale"?#
A scale is an object that gets attached to an axis. The class documentation
is at scale
. set_xscale
and set_yscale
set the scale on the respective Axis objects. You can determine the scale
on an axis with get_scale
:
fig, ax = plt.subplots(layout='constrained',
figsize=(3.2, 3))
ax.semilogy(x, x)
print(ax.xaxis.get_scale())
print(ax.yaxis.get_scale())
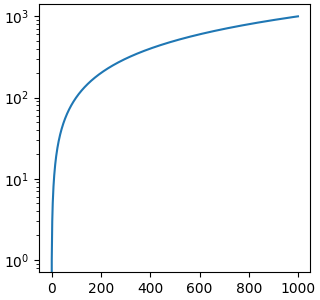
linear
log
Setting a scale does three things. First it defines a transform on the axis
that maps between data values to position along the axis. This transform can
be accessed via get_transform
:
print(ax.yaxis.get_transform())
LogTransform(base=10, nonpositive='clip')
Transforms on the axis are a relatively low-level concept, but is one of the
important roles played by set_scale
.
Setting the scale also sets default tick locators (ticker
) and tick
formatters appropriate for the scale. An axis with a 'log' scale has a
LogLocator
to pick ticks at decade intervals, and a
LogFormatter
to use scientific notation on the decades.
print('X axis')
print(ax.xaxis.get_major_locator())
print(ax.xaxis.get_major_formatter())
print('Y axis')
print(ax.yaxis.get_major_locator())
print(ax.yaxis.get_major_formatter())
X axis
<matplotlib.ticker.AutoLocator object at 0x7d8bb858bce0>
<matplotlib.ticker.ScalarFormatter object at 0x7d8bb8589190>
Y axis
<matplotlib.ticker.LogLocator object at 0x7d8bb858ba10>
<matplotlib.ticker.LogFormatterSciNotation object at 0x7d8bd324a8d0>
Total running time of the script: (0 minutes 5.609 seconds)